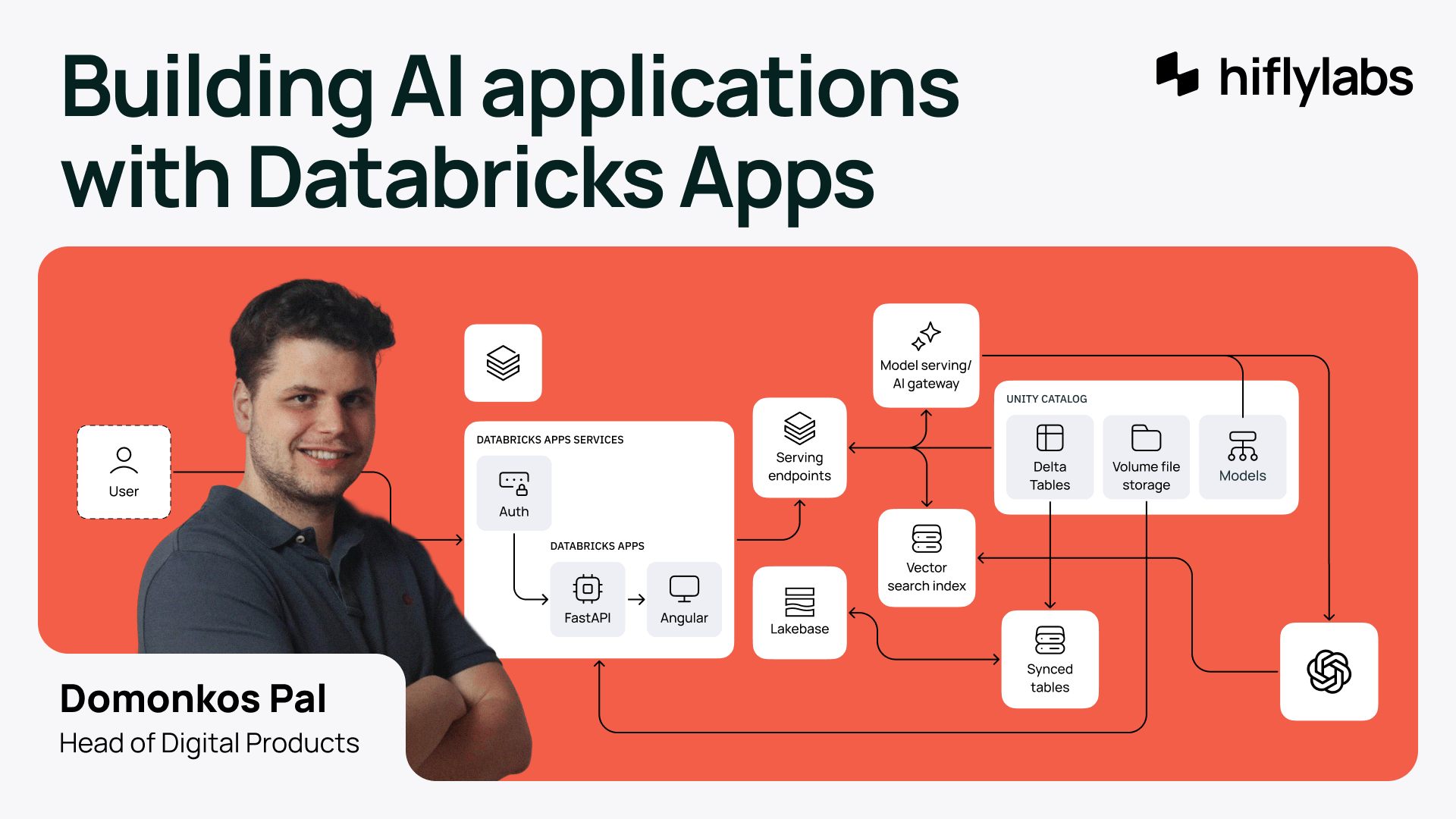
Building an AI Application with Databricks Apps in 30 Days
Discover how to build a production-ready AI application on Databricks Apps in just under a month. Learn from our journey, challenges, and architectural choices.
Reading time: 4 min
A data scientist needs to understand the broader business context into which his or her analysis fits and to be able to provide the right data-based answer to a proper question. The business relevance of his/her results and his/her solid methodological background together ensure that a project can create business value in the long run.
Most of our analytical projects do not have a data scientist on the client’s side, so it is our responsibility to put our results into an understandable form. In such cases, the one-time analyst has a particularly difficult task, as he/she also has to think like the business side. Ideally, the analyst and the client begin to cooperate at the beginning of the project: the analyst understands the value the project can create, and the client recognizes what information the analyst needs. Proper communication is so important that many times we dedicate an expert for it in the project. Eszter Somos wrote about this earlier on the blog (Analytics Translator – A geek on the one hand, and a brilliant communicator on the other, a therapist for business and IT).
I approach the subject from a slightly different perspective, as a classic analyst.
The main difference between business and IT people is that while the former thinks more of more abstract goals, the latter requires precise definitions for his/her work. In this respect, a data scientist is somewhere in between, but definitely far enough from the business side to make communication between the two a challenge. On the one hand, the analyst needs to understand the high-level business goal, but on the other hand, he/she also needs the details. Let’s take a simple example: a customer in charge of business development wants to predict the return on investment. For an analyst to get started, he/she needs to know exactly what return means, how to calculate the return on previous projects, and what information can be used to make a forecast.
At the same time, we cannot expect the client to think of everything that is needed for the analysis. Therefore, it is a good idea for the analyst to be aware of the business need as well, as this may remind them of additional issues or pitfalls, and they can make analytical decisions with complete certainty that would require business assistance failing a broader context.
As a general rule, we strive to deliver results that are as valuable as possible. These can be simple statistics at first, or quite particular results (hey, you produced a quite good sales result in 2018 in Borsod, you know why?).
As time goes on, we will introduce more and more complex analytical tools to explore the patterns, and so we will have an increasingly difficult time telling them. In such cases, we strike a sensible balance, where on one side we are trapped by complete incomprehension and on the other the unpleasant feeling of dishonesty caused by oversimplification. The whole picture always comes with a lot of assumptions, additions, and comments that all add to the truth, but obviously it doesn’t make sense to include the whole thing in a forty-five-minute presentation. While on the one hand, technical details need to be censored, and on the other hand, commercially important and useful information that seems methodically a by-product needs to be added. Thus, the long description of the cross-validation strategy is replaced by a diagram of the distribution of sales between countries. Therefore, we use the mean (absolute) error to measure the quality of modeling, which a statistician would certainly not use on his own. Ideally, there are several important methodological decisions behind each issue and the outcome of a final presentation.
Analytical projects will always have two end results: one that we show to the client and displays the results we find useful, and another that supports the results and includes the necessary technical details. Together, the two results ensure that a project can create business value in the long run. If the results for the business are great but the technical details are not right, the decisions based on the analysis will not be optimal. If the methodological background is ok but lacks business insights, the results will not be put into practice.
Dániel Szokolics - Data Scientist