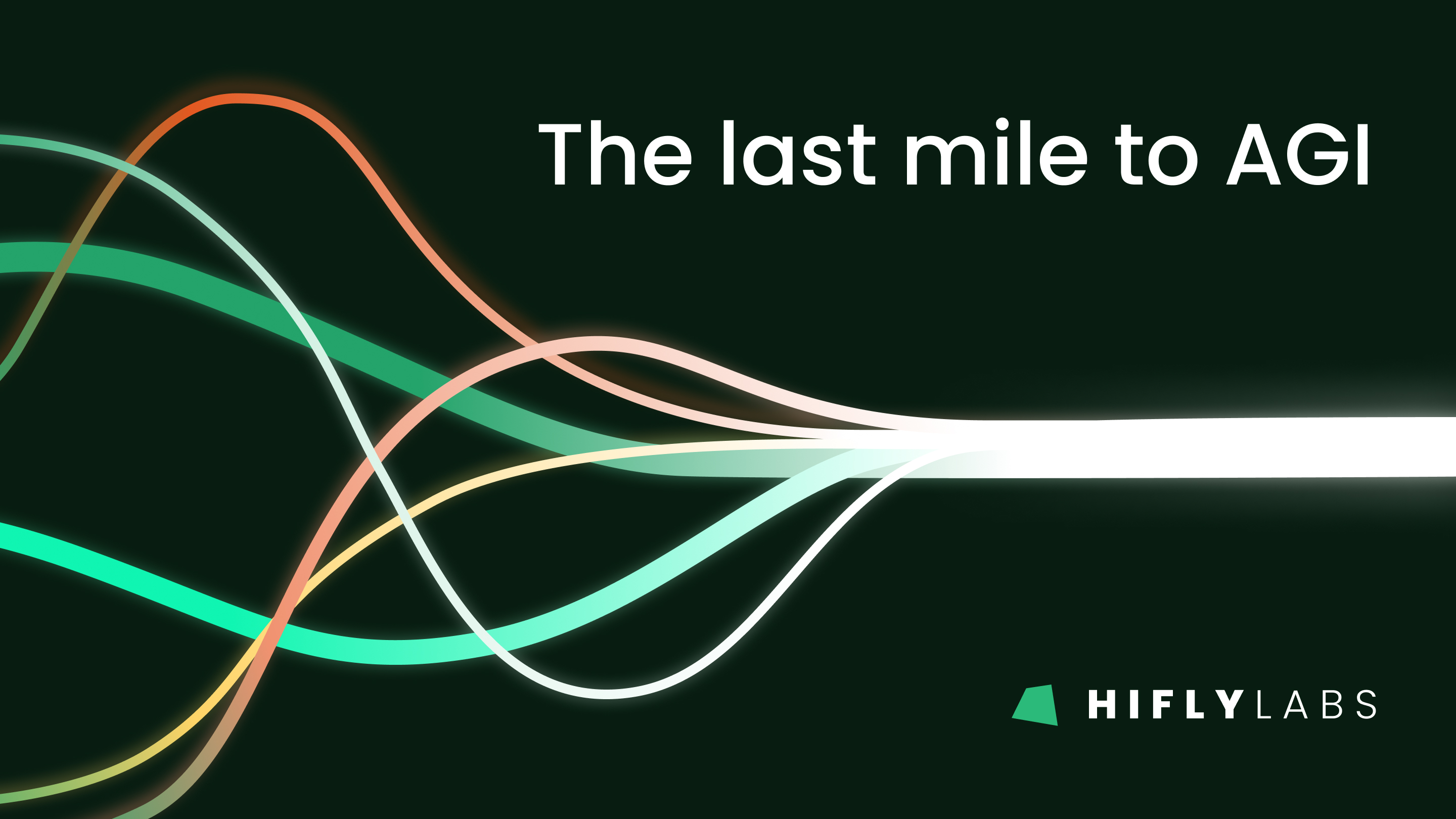
Path to AGI – Part 2
Comprehensive analysis of recent AI progress: reasoning models, embodied AI, and multimodality – all pointing toward the goal of general intelligence.
Scientific breakthroughs have dramatically extended human lifespans in the past century. Now, artificial intelligence stands to catalyze the next great leap in research, patient care, and longevity. While this revolution in healthcare and life sciences has immense potential, currently available technologies also present significant challenges.
Modeling on healthcare data houses major potential. Doing so allows us to make patient care more efficient, and move towards targeted prevention in the long run. This includes:
- Personalized care and population-wide analyses for epidemiology research
- More effective and efficient operative processes in patient care
- Involving patients in prevention and improving their experience with the system
- Clear and understandable lifestyle-related communication to incentivize positive change
- Organization and strategic reform of patient care
Let’s take a look at the current state of affairs!
To understand where we’re headed, we must first take a look at the state of the available data for healthcare professionals and scientific researchers:
Information is scattered through different subsystems. Even if it's already logged in a central database (e.g. in a hospital or even national repository) there is usually a wide variety of formats. Tabular data, scanned documents, handwritten notes, and medical imaging can be found next to each other. Unstructured inputs (up to 80-90 percent of all information) require cutting-edge technologies to consolidate.
As one of the most sensitive areas to work with, healthcare sets a high standard in data security and governance. Often, on-prem or local cloud solutions are required, and pulling all the relevant data into one place can be hindered by regulations.
Better understanding of available information leads to faster breakthroughs in all areas from research to patient care. Let’s take a look at the range of available data based on complexity!
As mentioned before, a good part of all the data available is “ready to use”. Such structured data, or documents that can be easily chunked and processed, are a great starting point. Lab reports, prescriptions, medical histories, and tables are such entries. Building highly algorithmic, easily validated pipelines in their case is possible even without generative AI.
A slightly more complex type of data comes from medical imaging—such as X-rays or MRI—or even handwritten text. Multimodal generative AI or visual deep learning solutions can quickly save time by selecting, processing, and sorting medical data, images, and documents according to different criteria.
The most complex, free-text documents (operative reports, discharge summaries, research documentation, etc.) contain 70-80% of the relevant information in an unstructured format, but they can now be analyzed and consolidated, with the advent of language models. In the long run, this is the biggest win for healthcare, as it benefits all stakeholders from research to pharmaceuticals and patient care.
Available data is just one step of the process, but how do we turn it into AI-driven healthcare? We believe that each industry has different use cases and potential rollout periods.
While pharma companies such as Moderna already use AI in their breakthroughs, other areas are on different timelines. Administrative support is easily validated and can be deployed almost as-is, once approved, multi-modal diagnosis will require extra care.
The next step in AI-supported healthcare is compound multi-agent systems. At Hiflylabs, we have built similar solutions before. These systems allow the coordination of multiple agents for complex tasks with great efficiency and accuracy. They’ve proven extremely useful when working with vast amounts of data. Similarly vast amounts of information lies in patient documentation and scientific literature, and extracting the value from them will very likely define the next few years of developments.
Here’s how we see industries benefiting from advances in tech!
We can supercharge the synthesization of all relevant medical information when creating new drugs, as well as in communication with industry stakeholders and patients.
Moderna, one of the largest pharmaceutical companies, has announced its partnership with OpenAI over 2 years ago. Over 3000 of their employees work with ChatGPT, and have over 750 fine-tuned versions as of 6 months ago, and even Moderna’s covid vaccine team used AI in their research. It allowed them to ready their vaccine for human trials in just 42 days, making them one of the key players in the fight against the disease.
The models help them across areas from research to clinical trials and compliance, allowing more efficient decision-making in processes such as dose optimization, drafting correspondence to regulators, and predicting enzyme structures that best suit manufacturing.
Communication and diagnostics are some of the key areas in patient care that can benefit the most from AI. Efficient and comprehensive communication ensures great doctor-patient relationships, while accurate diagnosis is the cornerstone of all healthcare.
New tools can alleviate the administrative burden of medical professionals. Scheduling, appointments, and notetaking can easily be introduced, allowing staff to focus on patients.
Diagnostic support is another area where AI can lead to breakthroughs. By processing imaging results, models are able to identify early signs of illnesses, leading to faster and better treatment across the board.
For example, Google’s Med-Gemini model, fine-tuned from Gemini, beat GPT-4 in every benchmark and was successful in assisting doctors in predictive analytics for more efficient preventive medicine. As a result, the model helped identify early signs of cancer, or even assist in real-time by confirming whether a surgeon removed all cancerous tissue.
Multi-modal diagnostics are the next major stepping stone for AI in healthcare. The tech already exists, developers are working on turning it into real-life use cases. By compiling and analyzing people’s medical records, lab results, discharge summaries and more, they will assist in identifying potential health risks before the first significant symptoms, and allowing for early intervention or even complete prevention.
Further down the line, personalized medicine will ensure that everyone receives the care and therapy they exactly need, considering their current condition, medical history and environment.
Multi-agent systems are able to handle vast amounts of written information, consolidate it into easy to interpret summaries, and extract specific key points in mere seconds. This has already proved useful in various use cases, and has the potential to reshape medical research as well.
These systems can find correlations in large datasets, with references to where different data points are taken from, making them extremely useful and easy to validate in academic research cases.
By virtue of having access to already existing knowledge, and paired up with their already existing conversational and generational qualities, AI/ML tools can also serve as a great way to enhance medical education.
AI’s role in the future of healthcare is supporting and complementary.
It is important that we don’t mix responsibility and accountability. AI systems can and should be held responsible for a narrow range of tasks they’re specifically designed for, but the final say in all included areas (from research to pharma and patient care) must fall on a trained professional.
Development, and especially adaptation of new tech will take time in an area as sensitive as healthcare. As developers, we have to focus on accuracy, as well as building trust with medical professionals.
AI developers need to create opportunities for validating and testing their products at an increasing scale, starting out with easily algorithmizable cases and building up to complex uses over time. They also have to provide enough data in an easy-to-understand and transparent format within a short time for doctors to come to conclusions, especially so in cases where multiple data sources are involved.
Devs must focus on creating tools that support diagnostics, alleviate staff of the burden of administrative work, and let them focus on the things that actually matter: healing people and saving lives.
Comprehensive analysis of recent AI progress: reasoning models, embodied AI, and multimodality – all pointing toward the goal of general intelligence.
Democratizing multi-agent systems by creating user-centric digital products around them requires an entirely new outlook on the development process. Find out how to tackle new challenges in frontend, backend, and UX/UI design!
Hiflylabs is your partner in building your future. Share your ideas and let's work together!