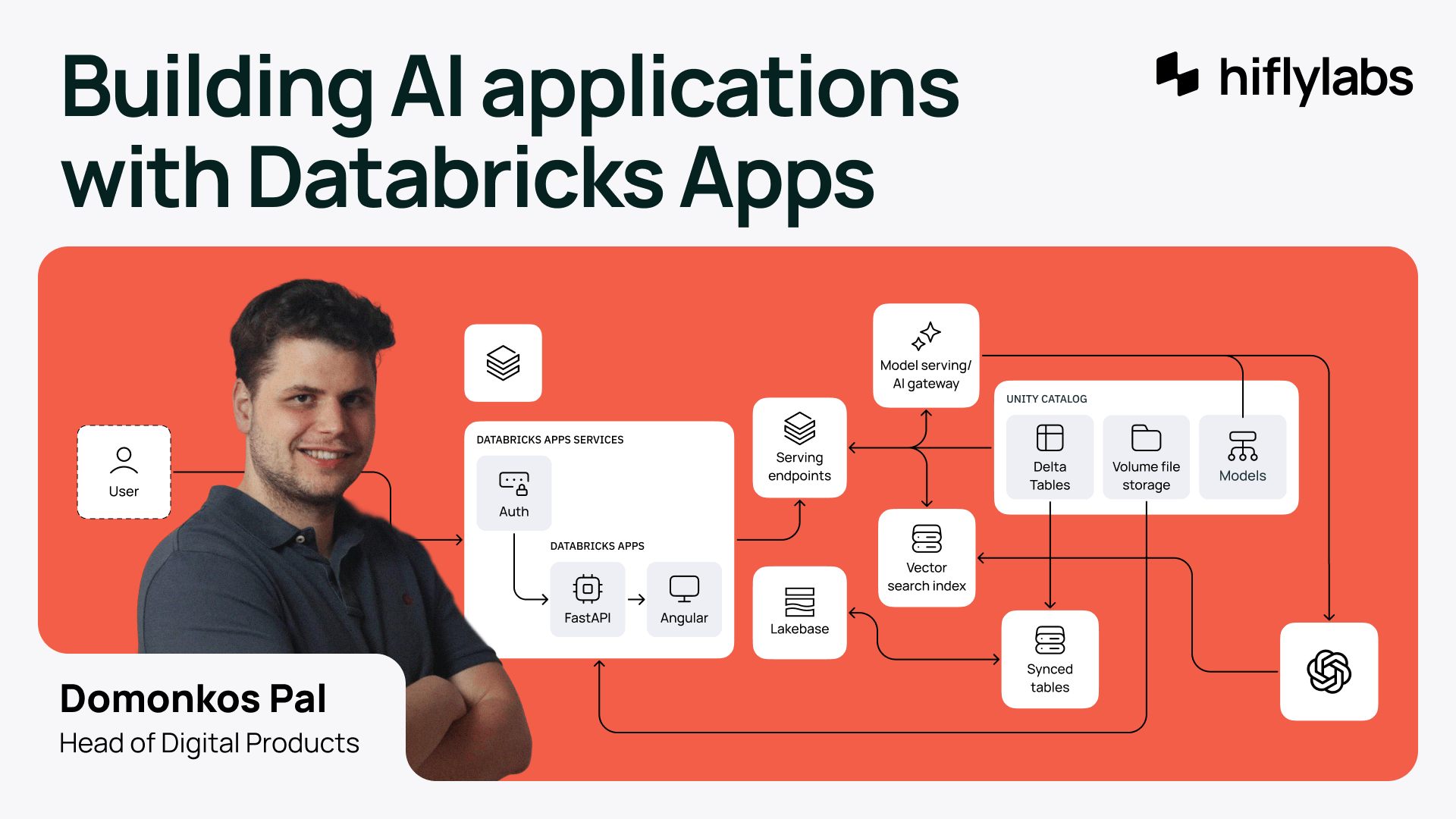
Building an AI Application with Databricks Apps in 30 Days
Discover how to build a production-ready AI application on Databricks Apps in just under a month. Learn from our journey, challenges, and architectural choices.
Explore the current state of Generative AI and the challenging road towards Artificial General Intelligence (AGI). We review key limitations, needed breakthroughs, and future scenarios.
As of August 2024, we find ourselves at a curious juncture. The initial surge of excitement surrounding Generative AI has begun to wane, yet beneath the calm, a new wave of innovation is boiling. This piece aims to explore the current state of AI, its practical applications, and the challenging road that lies ahead as we inch closer to Artificial General Intelligence (AGI).
The hype cycle of Generative AI has reached its nadir. ChatGPT, once the poster child of this AI revolution, has seen its user base dwindle below early 2023 levels.
However, this decline doesn't tell the whole story. Other models, particularly Claude and Gemini, have experienced significant growth, indicating a shift in user preferences rather than a wholesale rejection of the technology.
Several factors contribute to this apparent slowdown:
1. The last major advancement, GPT-4, was released in March 2023. Since then, progress has been incremental rather than revolutionary.
2. The limitations of GenAI, including hallucinations and other inherent flaws, have become apparent to a wider audience. Only a select few are exploring adequate workarounds.
3. Media outlets have been presenting training data scarcity as a potential showstopper for future progress.
However, in the corporate world, successful integration of AI technologies has grown continuously.
Businesses are increasingly identifying valuable applications across various industries and operations. Nvidia's recent financial results, which saw revenue more than double in the latest quarter, provide clear evidence that AI adoption remains robust.
Despite the current lull, several developments on the horizon promise to reignite interest and push the boundaries of what's possible:
1. The next generation of OpenAI’s GPT models (currently in development under codemanes like Strawberry and Orion) are expected to arrive by the end of the year. These are anticipated to be nearly the strongest possible with current methods, potentially surpassed only by projects like Microsoft's Stargate.
2. Models are becoming increasingly multimodal, trained on image, video, audio, and text simultaneously. The ability to switch between these modalities flexibly and in real-time is likely to usher in a new wave of excitement.
3. AI Agents are only beginning to mature, and will continue to drive process automation across every organization globally.
4. Major developers are working towards a breakthrough in generalized reasoning. This could solve issues related to hallucinations, formal logic, and math/coding abilities simultaneously, representing a significant step towards self-improving or completely autonomous models. Most industry experts predict this breakthrough between 2025-2028, with AGI potentially arriving by 2030.
Even if no further breakthroughs were made, the current trajectory of GenAI promises decades of innovation through incremental advances:
Any-to-any multimodality
These are a given. However, meaningful progress is unlikely to stagnate. Inherent limitations of current GenAI architectures are clearly defined and being actively worked on.
Let's review the missing pieces.
To understand the path to AGI, we must first recognize the limitations of our current models:
Static parameters, no real memory or continuous learning/retraining
Most people have expected LLMs to not have any of these limitations in the first place and were thoroughly disappointed when they encountered them. Many of our current GenAI projects involve building around and solving these problems in roundabout ways.
Even researchers hoped that sheer scaling, bigger and bigger models would bring us closer to solving these capabilities.
Recent concerns about running out of novel training data have been amplified by articles suggesting that AI models fed AI-generated data quickly produce nonsense. However, this oversimplifies a complex issue.
Model collapse occurs only when the training corpus consists exclusively of low-quality data, regardless of whether it's synthetic or organic. High-quality, diverse datasets are the key for optimal results. In fact, combining high-quality synthetic data with high-quality organic data yields better results than using either exclusively. Dario Amodei, CEO of Anthropic, has proposed that by injecting high quality generated data into organic datasets, we can create unlimited training engines.
Still, some experts are worried, because training data requirements for improving multimodal capabilities grows exponentially, while gains in model performance scales logarithmically.
Should we reach the future autonomous agents that are capable of dynamic learning from their environments, we could utilize a continuous stream of new information as training data, which nullifies the above concerns. This is the homerun all big developers are aiming for.
Now that we’ve put this issue to bed, let’s review the innovations needed for general intelligence, addressing all concerns we’ve discussed so far!
Scaling is not all we need. Important ingredients are missing from today’s architectures.
To gain deeper insights into these challenges, let's turn to one of the leading voices in AI.
Andrej Karpathy was one of OpenAI’s co-founders, has formerly led the Tesla Autopilot computer vision team, and is nowadays doing exemplary AI education work. He makes video guides, posts on social media frequently openly discussing hard problems of his field, and has recently founded a new company dedicated to knowledge transfer. So I will cite him as a primary source for some important ideas here.
Let’s start with a more mundane limitation.
Karpathy has highlighted tokenization as a current limitation of language models. This seemingly mundane aspect of text processing is at the root of many issues, including:
Difficulty in spelling words correctly
Inability to perform simple string processing tasks
Poorer performance in non-English languages
Struggles with simple arithmetic
Difficulties in coding tasks
For instance, the infamous "strawberry problem" - where models consistently fail to count the number of 'r's in "strawberry" - is a direct result of tokenization. The word is usually represented as two tokens, making it architecturally challenging for models to count individual characters without resorting to roundabout problem-solving methods.
But this is an issue that we expect to be fixed rather easily, compared to others.
A significant obstacle in the path of GenAI becoming AGI is that existing generative models heavily rely on human input during training—this is called Reinforcement Learning from Human Feedback (RLHF). Truly superhuman capability was only achieved so far through models learning by themselves, self-play, a method successfully employed in narrow domains like DeepMind's AlphaZero for chess and Go—this is called Reinforcement Learning (RL).
The challenge lies in creating a model that can learn everything by itself to reach superhuman capability AND achieve generalization simultaneously. This is difficult for two main reasons:
1. The search space for the real world is enormous, making a pure RL or pure self-play training approach computationally infeasible at present.
2. The reward function for general intelligence is complex and dynamic. While it's relatively straightforward to create a perfect model for winning chess, creating a model capable of winning all possible games requires the ability to dynamically set new goals and retrain on the fly according to varying rules and requirements.
Andrej Karpathy explains the problem of RL vs RLHF more adequately.
Let’s review how researchers are exploring novel approaches to tackle this fundamental challenge.
Researchers are exploring novel approaches to tackle this fundamental challenge. The Alberta Plan, for instance, proposes a radical rethink of AI development:
Because accurate, all encompassing knowledge of the world's state or transition dynamics is impossible, it follows that continual learning and adaptation is necessary.
Now that we’ve seen how continual and meta-learning (learning to learn) are naturally linked, let’s conclude by looking at possible trajectories of progress.
Whether we achieve AGI in the near future or continue on a path of incremental advances, we are undoubtedly living in an era of unprecedented technological progress. The challenges we face in developing more advanced AI systems are complex, but even our current technological maturity presents exciting opportunities for innovation.
Ethan Mollick has recently proposed 4 possible future scenarios for AI:
From today’s perspective, either a slower or a more exponential progress scenario still seems equally likely. But I disagree that a slower rate would equal hitting a wall.
GenAI has just begun to revolutionize the Healthcare & Life Sciences sector, providing never before seen possibilities in predictive diagnostics and new ways of drug discovery. We could propel endless innovation just productizing and ripening our current level of AI.
But if we continue down the path of progress any faster, it's critical to consider not just the technological aspects but also the broader implications. We currently lack societal guardrails for a radically changing global economy and proper frameworks for dealing with potential artificial consciousness. As Leopold Aschenbrenner discusses in his essay "Situational Awareness", we may be ill-equipped for a fast takeoff scenario.